ORLANDO – Imagine having to work through the complex administrative bottlenecks of juggling roughly 1,300 patients in circulation each day at a 1,000-bed hospital.
As an early adopter of using AI in healthcare, Tampa General Hospital uses data and machine learning in a practical sense. And not in the cool, powerful, big data way you might think.
Machine learning and AI help keep patients moving smoothly through the hospital’s administrative processes, such as dietary needs and testing.
“Our view is a very practical application of AI. The truth is, we really haven’t yet fully unlocked our data in healthcare,” said Scott Arnold, executive vice president and chief information officer at TGH.
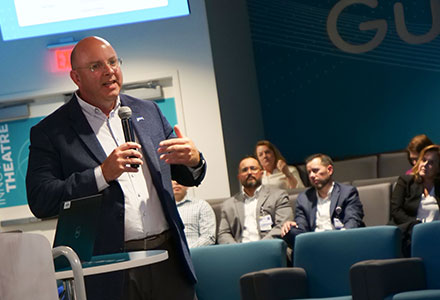
Arnold was one of the handful of speakers and panelists at the inaugural Data and AI for Health and Wellness: Best Practices Forum hosted by Florida Blue/GuideWell and the University of South Florida Muma College of Business.
“The challenge is really unlocking the data that we’re sitting on. What’s in front of us is really using machine learning and AI for insights that is practical for clinicians in their space. You’ve got to put it in the workflow of the clinicians,” he said.
About 110 policy makers, healthcare industry leaders, academics, and students attended the one-day event focused on using AI and data in healthcare. It was held Jan. 26 at the GuideWell Innovation Center in Orlando.
The purpose of the conference was to bring together industry leaders and academics to share ideas, promote collaboration and offer best practices on the use of data and AI in the healthcare industry.
The event was the first in what organizers say will be a series of collaborative conferences related to AI and data between USF and Florida Blue. USF’s Center for Analytics and Creativity helped organize the conference.
The forum featured four keynote speakers and a panel discussion where ChatGPT, a chatbot app, was a surprise guest.
One of the speakers were Soumya Sen, an associate professor and McKnight Presidential Fellow in the Carlson School of Management at the University of Minnesota.
Sen talked about his work on the COVID-19 Hospitalization Tracking project, which had received extensive media coverage for its collaborative and timely effort. The project was an example of how machine learning and AI was used to give the public valuable and up-to-date information.
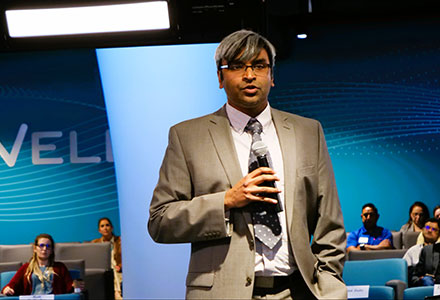
“This was an attempt to inform the public about the trends,” he said, adding that they had an army of undergraduates who helped process the data.
Students would check in with the health departments on the state and federal levels to collect the data. They also cross-checked and analyzed the data before adding it to the dashboard.
Another speaker, Alexander Fleiss, the CEO, chairman and co-founder of Rebellion Research, discussed the use of AI and deep learning in healthcare. He said AI in healthcare holds promise but is in the early stages. He likened managing AI in healthcare to managing children in a pre-K classroom.
“AI will be the mechanism that will bring about true healthcare affordability in America at any level,” he said. “Healthcare and AI’s issue is that the data is too murky to rely on.”
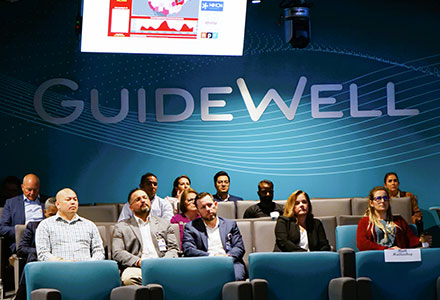
For example, research shows that machine learning could be used to screen for colorectal cancer and breast cancer, but those uses are extremely limited and highly inaccurate, because most health data is not clean enough, he said.
USF’s Kaushik Dutta, director of the School of Information Systems and Management, and Matthew Mullarkey, a professor of instruction and the director of the DBA Program, were both part of the panel discussion and shared important perspectives about big data and mobile health as well as ideas about education in the healthcare space.
To end the conference, organizers introduced ChatGPT as a panelist and asked the chatbot to explain the opportunities and challenges for AI in healthcare.
The chatbot's response: "The biggest opportunity for AI in healthcare is the potential for it to improve patient outcomes and efficiency of healthcare delivery by analyzing large amounts of data and making accurate predictions or identifying patterns that may not be apparent to human analysts."
"The biggest challenge is the complexity and variability of healthcare data, as well as the need for AI systems to be able to explain their decisions and be transparent in their operation to gain the trust of healthcare professionals and patients."
"Additionally, there are ethical concerns around the use of AI in healthcare, such as ensuring that AI systems do not perpetuate existing biases or discriminate against certain groups of patients.”